How to Convert Parquet to JSON in Python
Grace Collins
Solutions Engineer · Leapcell
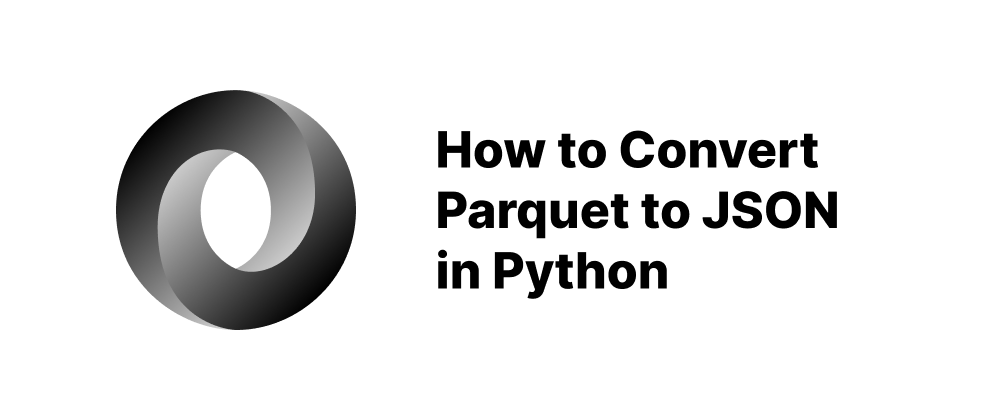
Key Takeaways
- Python libraries like Pandas and PyArrow simplify Parquet-to-JSON conversion.
- Chunked processing is essential for handling large Parquet files efficiently.
- Choosing the right engine (PyArrow, FastParquet, or DuckDB) impacts performance and compatibility.
Parquet is a columnar storage format optimized for performance and storage efficiency, widely used in big data processing frameworks like Apache Spark and Hadoop. JSON (JavaScript Object Notation), on the other hand, is a lightweight and human-readable format commonly used for data interchange. Converting Parquet files to JSON can be essential when sharing data with systems that prefer JSON or for easier data inspection.
This guide will demonstrate how to perform this conversion using Python, covering various methods suitable for different scenarios.
Prerequisites
Before proceeding, ensure you have the necessary Python libraries installed. You can install them using pip:
pip install pandas pyarrow fastparquet
pandas
: For data manipulation and analysis.pyarrow
: Provides Python bindings for Apache Arrow and is used for reading Parquet files.fastparquet
: An alternative Parquet reader and writer.
Method 1: Using Pandas and PyArrow
This is the most straightforward method, suitable for small to medium-sized Parquet files.
import pandas as pd # Read the Parquet file df = pd.read_parquet('data.parquet', engine='pyarrow') # Convert DataFrame to JSON and save to a file df.to_json('data.json', orient='records', lines=True)
orient='records'
: Each line of the JSON file corresponds to a row in the DataFrame.lines=True
: Writes the JSON file in a line-delimited format, which is useful for streaming and large files.
Method 2: Processing Large Files in Chunks
For large Parquet files that may not fit into memory, processing the data in chunks is advisable.
import pyarrow.parquet as pq import json # Open the Parquet file parquet_file = pq.ParquetFile('large_data.parquet') # Process and write in chunks with open('large_data.json', 'w') as json_file: json_file.write('[') # Start of JSON array first_chunk = True for batch in parquet_file.iter_batches(batch_size=10000): df_chunk = batch.to_pandas() json_str = df_chunk.to_json(orient='records') json_str = json_str[1:-1] # Remove the surrounding brackets if not first_chunk: json_file.write(',') else: first_chunk = False json_file.write(json_str) json_file.write(']') # End of JSON array
This approach reads the Parquet file in batches, converting each batch to JSON and writing it to the output file incrementally, thus managing memory usage efficiently.
Method 3: Converting Specific Columns
If you only need certain columns from the Parquet file, you can specify them during the read operation.
import pandas as pd # Read specific columns from the Parquet file df = pd.read_parquet('data.parquet', columns=['column1', 'column2']) # Convert to JSON df.to_json('selected_columns.json', orient='records', lines=True)
This method reduces memory usage and processing time by only loading the necessary data.
Method 4: Using FastParquet
fastparquet
is an alternative to pyarrow
for reading Parquet files and may offer performance benefits in certain scenarios.
import pandas as pd # Read the Parquet file using fastparquet df = pd.read_parquet('data.parquet', engine='fastparquet') # Convert to JSON df.to_json('data_fastparquet.json', orient='records', lines=True)
Choose the engine that best suits your performance and compatibility requirements.
Method 5: Using DuckDB
DuckDB is an in-process SQL OLAP database management system that can efficiently handle Parquet files.
import duckdb # Convert Parquet to JSON using DuckDB duckdb.sql(""" COPY (SELECT * FROM 'data.parquet') TO 'data.json' (FORMAT 'json') """)
This method is particularly useful for complex queries and transformations during the conversion process.
Tips and Best Practices
- Handling Nested Data: Parquet files can contain nested data structures. Ensure that your JSON output maintains the desired structure. You may need to process nested fields accordingly.
- Data Types: Be aware of data type conversions between Parquet and JSON. Some types may not have direct equivalents and could require custom handling.
- Performance: For very large datasets, consider using chunked processing or tools optimized for big data to prevent memory issues.
Conclusion
Converting Parquet files to JSON in Python is achievable through various methods, each suited to different scenarios and requirements. Whether you're dealing with small files or large datasets, Python's rich ecosystem provides the tools necessary for efficient and effective data conversion.
Choose the method that aligns with your specific needs, considering factors like file size, performance, and data complexity.
FAQs
Use Pandas with PyArrow and to_json()
for a quick conversion.
Read and process the Parquet file in batches to manage memory usage.
Yes, use Pandas' columns
parameter to load only needed columns.
We are Leapcell, your top choice for hosting backend projects.
Leapcell is the Next-Gen Serverless Platform for Web Hosting, Async Tasks, and Redis:
Multi-Language Support
- Develop with Node.js, Python, Go, or Rust.
Deploy unlimited projects for free
- pay only for usage — no requests, no charges.
Unbeatable Cost Efficiency
- Pay-as-you-go with no idle charges.
- Example: $25 supports 6.94M requests at a 60ms average response time.
Streamlined Developer Experience
- Intuitive UI for effortless setup.
- Fully automated CI/CD pipelines and GitOps integration.
- Real-time metrics and logging for actionable insights.
Effortless Scalability and High Performance
- Auto-scaling to handle high concurrency with ease.
- Zero operational overhead — just focus on building.
Explore more in the Documentation!
Follow us on X: @LeapcellHQ