How to Flatten a List of Lists in Python
Daniel Hayes
Full-Stack Engineer · Leapcell
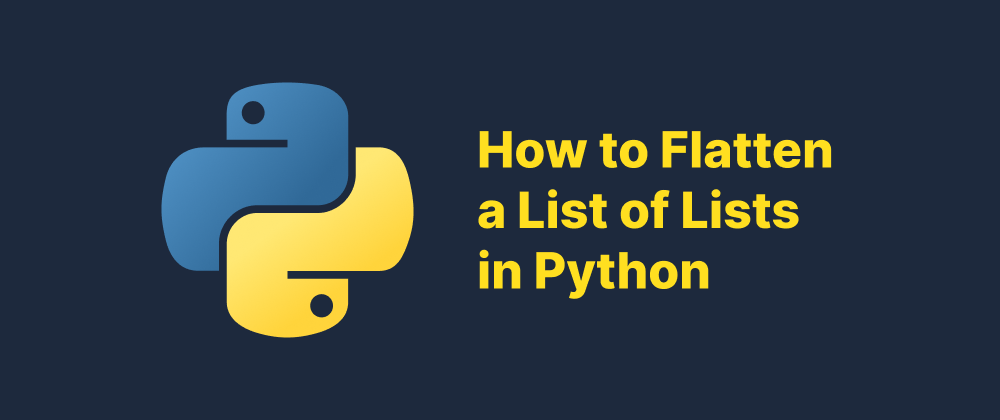
Key Takeaways
- Using list comprehension or
itertools.chain()
is the most concise and efficient approach. flatten_recursive()
can handle lists with arbitrary levels of nesting.- The choice of method should depend on the list structure and performance requirements.
Flattening a list of lists in Python involves converting a nested list structure into a single, one-dimensional list. This is a common task when dealing with data stored in nested lists, such as matrices or JSON data. Below are several methods to achieve this, each with its own advantages.
1. Using a For Loop with .extend()
This method involves iterating through each sublist and extending a new list with the elements of each sublist.
def flatten_extend(nested_list): flat_list = [] for sublist in nested_list: flat_list.extend(sublist) return flat_list
This approach is straightforward and readable, making it suitable for beginners.
2. Using List Comprehension
List comprehension provides a concise way to flatten a list of lists.
def flatten_comprehension(nested_list): return [item for sublist in nested_list for item in sublist]
This one-liner is efficient and widely used in Pythonic codebases.
3. Using itertools.chain()
The itertools
module offers the chain()
function, which can be used to flatten a list of lists.
import itertools def flatten_chain(nested_list): return list(itertools.chain.from_iterable(nested_list))
This method is efficient and suitable for large datasets, as it avoids the overhead of nested loops.
4. Using functools.reduce()
The reduce()
function from the functools
module can concatenate sublists into a single list.
from functools import reduce from operator import add def flatten_reduce(nested_list): return reduce(add, nested_list, [])
While this approach works, it's generally less readable and not recommended for simple flattening tasks.
5. Using sum()
The built-in sum()
function can also flatten a list of lists by specifying an empty list as the starting value.
def flatten_sum(nested_list): return sum(nested_list, [])
This method is concise but may be less efficient for large lists due to the way sum()
handles list concatenation.
6. Using Recursion for Deeply Nested Lists
For lists with arbitrary levels of nesting, a recursive approach can be used.
def flatten_recursive(nested_list): flat_list = [] for item in nested_list: if isinstance(item, list): flat_list.extend(flatten_recursive(item)) else: flat_list.append(item) return flat_list
This method handles nested lists of any depth but should be used with caution to avoid maximum recursion depth errors.
7. Using NumPy for Numerical Data
If you're working with numerical data, NumPy provides efficient methods to flatten arrays.
import numpy as np def flatten_numpy(nested_list): return np.array(nested_list).flatten().tolist()
This approach is highly efficient for numerical computations and is commonly used in data science applications.
Conclusion
Each method has its own use cases:
- For Loop and List Comprehension: Best for readability and simplicity.
itertools.chain()
: Efficient for large datasets.reduce()
andsum()
: Concise but may have performance drawbacks.- Recursion: Suitable for deeply nested lists.
- NumPy: Ideal for numerical data processing.
Choose the method that best fits your specific needs and the nature of your data.
FAQs
Use list comprehension: [item for sublist in nested_list for item in sublist]
.
Only the recursive method (flatten_recursive
) can handle arbitrary-depth nesting.
Use NumPy when dealing with numerical data for better performance.
We are Leapcell, your top choice for hosting Python projects.
Leapcell is the Next-Gen Serverless Platform for Web Hosting, Async Tasks, and Redis:
Multi-Language Support
- Develop with Node.js, Python, Go, or Rust.
Deploy unlimited projects for free
- pay only for usage — no requests, no charges.
Unbeatable Cost Efficiency
- Pay-as-you-go with no idle charges.
- Example: $25 supports 6.94M requests at a 60ms average response time.
Streamlined Developer Experience
- Intuitive UI for effortless setup.
- Fully automated CI/CD pipelines and GitOps integration.
- Real-time metrics and logging for actionable insights.
Effortless Scalability and High Performance
- Auto-scaling to handle high concurrency with ease.
- Zero operational overhead — just focus on building.
Explore more in the Documentation!
Follow us on X: @LeapcellHQ