How to Perform Matrix Multiplication in Python
Grace Collins
Solutions Engineer · Leapcell
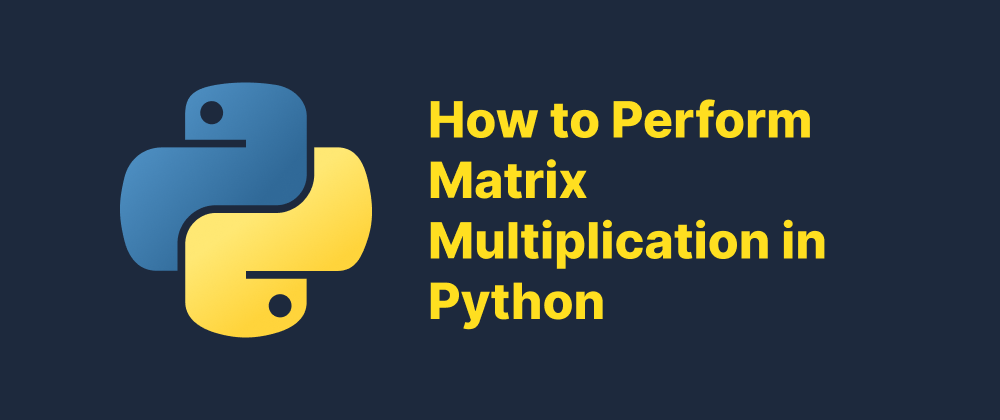
Key Takeaways
- NumPy provides efficient and concise tools for matrix multiplication.
- The
@
operator is a clean, modern syntax for matrix multiplication. - Valid matrix dimensions are essential for successful multiplication.
Matrix multiplication is a fundamental operation in linear algebra with wide applications in fields such as data science, machine learning, computer graphics, and scientific computing. Python offers several methods and libraries to perform matrix multiplication efficiently and accurately.
This article will guide you through different ways to perform matrix multiplication in Python, from basic list comprehension to using powerful libraries like NumPy.
1. Matrix Multiplication Basics
Matrix multiplication is not the same as element-wise multiplication. To multiply two matrices A and B, the number of columns in A must match the number of rows in B. The resulting matrix C will have the same number of rows as A and the same number of columns as B.
2. Using Nested Loops (Pure Python)
The most straightforward way to multiply two matrices is by using nested loops.
def matrix_multiply(A, B): result = [[0 for _ in range(len(B[0]))] for _ in range(len(A))] for i in range(len(A)): for j in range(len(B[0])): for k in range(len(B)): result[i][j] += A[i][k] * B[k][j] return result # Example A = [[1, 2], [3, 4]] B = [[5, 6], [7, 8]] print(matrix_multiply(A, B)) # Output: [[19, 22], [43, 50]]
While this approach works, it’s not efficient for large matrices and is more prone to bugs.
3. Using NumPy's dot()
Function
NumPy is a popular Python library for numerical computing. Its dot()
function allows fast and concise matrix multiplication.
import numpy as np A = np.array([[1, 2], [3, 4]]) B = np.array([[5, 6], [7, 8]]) C = np.dot(A, B) print(C) # Output: [[19 22] # [43 50]]
This method is both efficient and readable, making it a preferred choice in most cases.
4. Using the @
Operator
In Python 3.5 and above, the @
operator is introduced as a convenient syntax for matrix multiplication.
import numpy as np A = np.array([[1, 2], [3, 4]]) B = np.array([[5, 6], [7, 8]]) C = A @ B print(C) # Output: [[19 22] # [43 50]]
This operator is equivalent to np.matmul()
or np.dot()
and is ideal for writing clean and concise code.
5. Using NumPy's matmul()
Function
matmul()
behaves similarly to dot()
, but with better support for broadcasting and higher-dimensional arrays.
import numpy as np A = np.array([[1, 2], [3, 4]]) B = np.array([[5, 6], [7, 8]]) C = np.matmul(A, B) print(C) # Output: [[19 22] # [43 50]]
Use matmul()
when dealing with tensors or batches of matrices.
6. Best Practices
- Prefer NumPy over pure Python loops for performance.
- Use the
@
operator ornp.matmul()
for readability. - Always validate the shape compatibility of matrices before multiplication.
Conclusion
Matrix multiplication in Python can be done using basic loops or more efficient tools like NumPy. For most real-world applications, using NumPy’s built-in functions or the @
operator is the most effective and reliable approach.
Whether you're doing basic linear algebra or building machine learning models, mastering matrix operations is essential — and Python makes it both accessible and powerful.
FAQs
Both perform matrix multiplication, but @
is cleaner and more readable in Python 3.5+.
NumPy is optimized for performance and reduces code complexity.
No, the number of columns in the first matrix must match the number of rows in the second.
We are Leapcell, your top choice for hosting Python projects.
Leapcell is the Next-Gen Serverless Platform for Web Hosting, Async Tasks, and Redis:
Multi-Language Support
- Develop with Node.js, Python, Go, or Rust.
Deploy unlimited projects for free
- pay only for usage — no requests, no charges.
Unbeatable Cost Efficiency
- Pay-as-you-go with no idle charges.
- Example: $25 supports 6.94M requests at a 60ms average response time.
Streamlined Developer Experience
- Intuitive UI for effortless setup.
- Fully automated CI/CD pipelines and GitOps integration.
- Real-time metrics and logging for actionable insights.
Effortless Scalability and High Performance
- Auto-scaling to handle high concurrency with ease.
- Zero operational overhead — just focus on building.
Explore more in the Documentation!
Follow us on X: @LeapcellHQ